

Next, we will load the dataset into a Pandas dataframe and change the current label names ( 0 and 1) to a more human-readable ones ( negative and positive) and use them for model training. On the worst revenge-of-the-nerds clichés the filmmakers could dredge up Remains utterly satisfied to remain the same throughout That loves its characters and communicates something rather beautiful about human nature Hide new secretions from the parental units label=0 means negative, label=1 means positive. Here are the first 5 lines of the training dataset. The only difference between TSV and CSV is that TSV uses a tab \t character as its delimiter instead of a comma, in the CSV format. The SST-2 dataset is stored in TSV format.
WORD WRITER MACHINE MOVIE
The dataset has two classes: positive and negative movie reviews. It contains 67,349 movie reviews for training and 872 movie reviews for testing. In this tutorial, we will use the SST-2 (Stanford Sentiment Treebank) which is one of the tasks in the GLUE benchmark. import numpy as npįrom tflite_model_maker import model_specįrom tflite_model_maker import text_classifierįrom tflite_model_nfig import ExportFormatįrom tflite_model_maker.text_classifier import AverageWordVecSpecįrom tflite_model_maker.text_classifier import DataLoader
WORD WRITER MACHINE INSTALL
sudo apt -y install libportaudio2 pip install -q tflite-model-maker-nightly To run this example, install the required packages, including the Model Maker package from the GitHub repo.

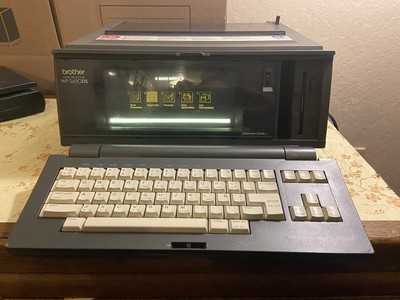
Prerequisites Install the required packages The dataset used in this tutorial are positive and negative movie reviews. The inputs should be preprocessed text and the outputs are the probabilities of the categories. The text classification model classifies text into predefined categories. This notebook shows an end-to-end example that utilizes the Model Maker library to illustrate the adaptation and conversion of a commonly-used text classification model to classify movie reviews on a mobile device. The TensorFlow Lite Model Maker library simplifies the process of adapting and converting a TensorFlow model to particular input data when deploying this model for on-device ML applications.
